Peter Norvig
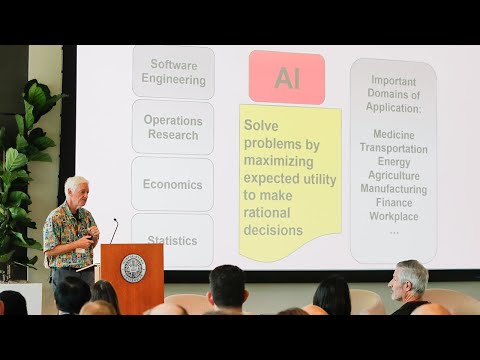
Peter Norvig (Google Director of Research) - What is Human-Centered AI? | Institute for Experiential AI (Nov 2023)
AI's evolution involves challenges in defining objectives, incorporating societal values, and addressing ethical concerns while achieving accuracy and mitigating unintended consequences. AI's impact on various fields, including justice, advertising, and creative industries, demands a responsible AI agenda that considers societal implications and diverse perspectives.
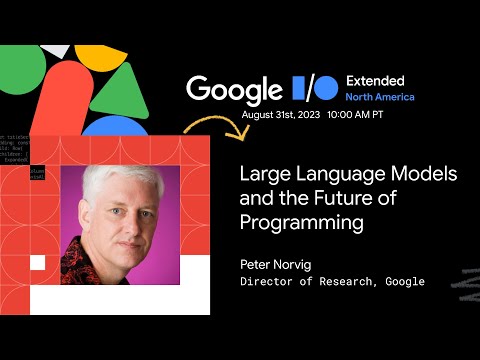
Peter Norvig (Google Director of Research) - Large Language Models and the Future of Programming | Google Developer Communities North America (Sep 2023)
The evolution of programming has shifted from traditional methods to probabilistic approaches, embracing AI collaboration for faster problem-solving and improved responsiveness. AI's role extends beyond code generation to encompass all stages of software development, necessitating continuous learning, adaptation, and ethical considerations for responsible AI adoption.
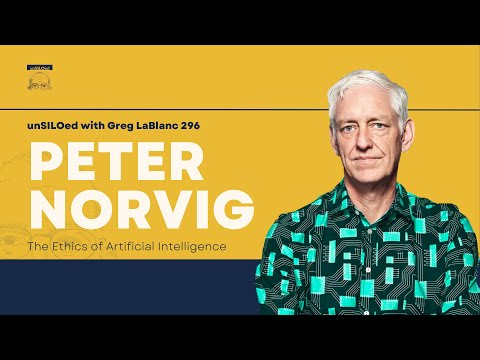
Peter Norvig (Google Director Of Research / Stanford) - Ethics Of AI (Jun 2023)
Peter Norvig emphasized the relationship between data science, AI, and machine learning, illustrating the shift from rule-based systems to data-driven models and end-to-end solutions.
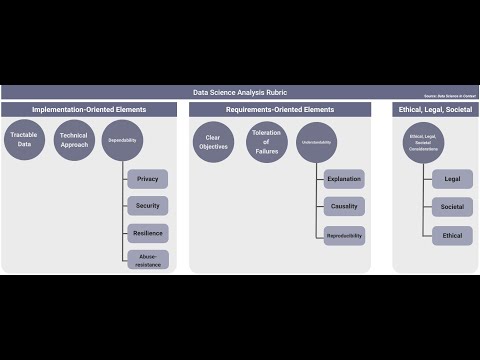
Peter Norvig (Google Director of Research) - Data Exchange Podcast on book, Data Science in Context (Jan 2023)
Data science education should emphasize problem-solving skills and de-emphasize coding, while balancing rigor and agility in projects through software engineering rigor. Ethical considerations, societal impacts, and legal aspects should be integral to data science practices and policies.
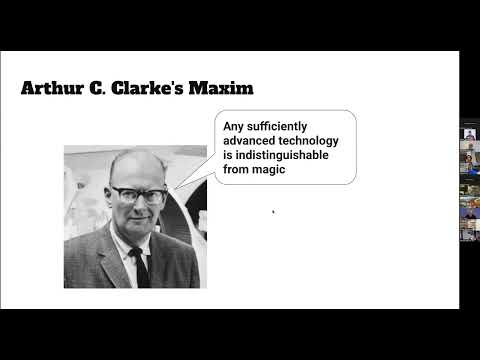
Peter Norvig (Google Director of Research) - Programming with Machine Learning (Dec 2022)
Software engineering is shifting from a logical approach to an empirical science, and AI problems require distinct techniques due to continuous change and uncertainty. Machine learning is becoming integrated throughout the software engineering lifecycle, offering potential solutions to problems beyond traditional techniques.
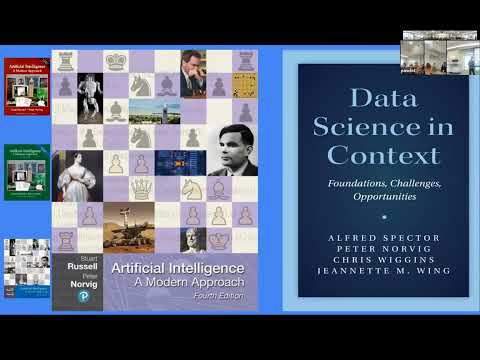
Peter Norvig (Google Director of Research) - Education For AI and By AI | Stanford (Oct 2022)
AI education should prioritize ethical principles and participation, and extend beyond algorithm implementation. AI's societal impacts and ethical challenges demand thoughtful considerations, particularly in sensitive areas like parole decisions and complex systems like self-driving cars.
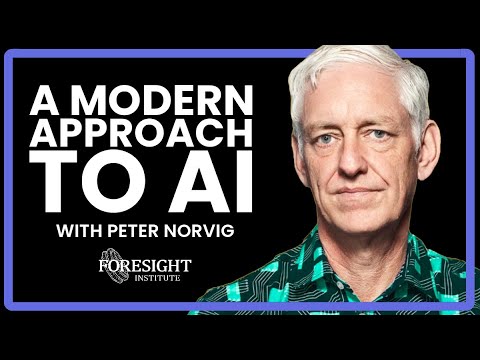
Peter Norvig (Google Director of Research) - Modern Approach to AI (Oct 2021)
AI development may follow a centralized or decentralized trajectory, and understanding and controlling AI systems pose challenges due to their complexity. Incorruptible autonomous systems and human control are crucial for mitigating societal risks and ensuring AI alignment with human values.
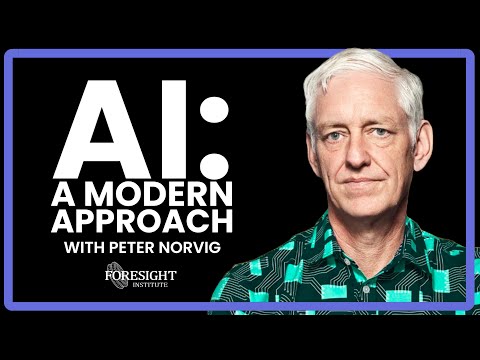
Peter Norvig (Google Director of Research) - AI (Oct 2021)
AI education has shifted from algorithm analysis to applications, focusing on societal impacts like ethics and privacy. AI's practical applications are growing, but concerns about unintended consequences and safety remain.
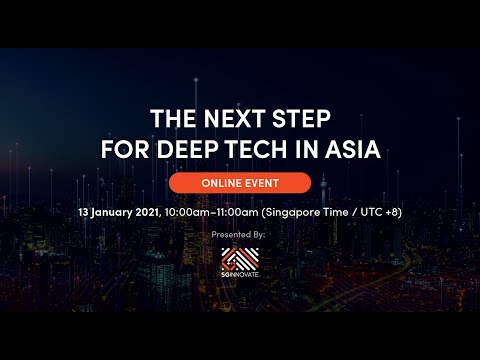
Peter Norvig (Google Director of Research) - The Next Step for Deep Tech in Asia (Jan 2021)
Deep tech innovations in AI, robotics, and quantum computing are reshaping industries and driving global collaboration, while commercialization challenges and talent development remain key considerations. Automation and robotics have accelerated due to the pandemic, creating opportunities but also requiring shorter sales cycles and wider customer targeting.
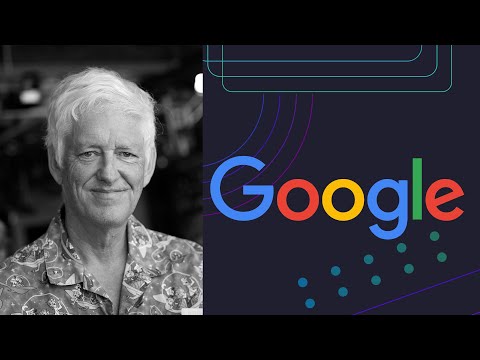
Peter Norvig (Google Director of Research) - Singularity is in the Eyes of the Beholder (Nov 2020)
AI has evolved from complex rules to probabilistic programming and impacted various aspects of society, presenting both opportunities and challenges. Norvig's insights emphasize gradual AI integration, responsible development, and continuous learning in this ever-evolving field.
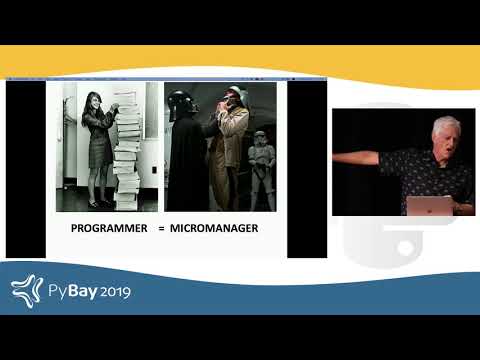
Peter Norvig (Google Director of Research) - As We May Program (Oct 2019)
AI in software development is evolving towards a data-driven, empirical approach, with ethical considerations and a focus on democratizing access. AI advancements should align with human needs, societal values, and global well-being.
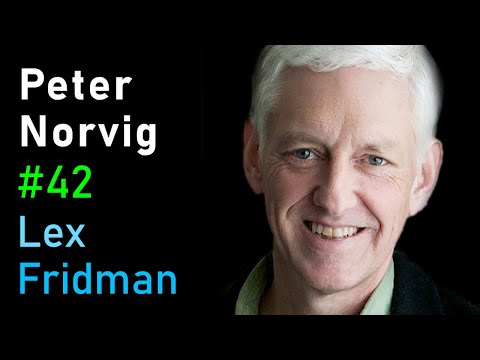
Peter Norvig (Google Director of Research) - Artificial Intelligence (Sep 2019)
AI's evolution from Boolean logic to machine learning, coupled with challenges in defining utility functions, necessitates a more nuanced approach to AI's societal impact. AI's future lies in integrating deep learning with traditional AI techniques, emphasizing explainability, trust, and validation.
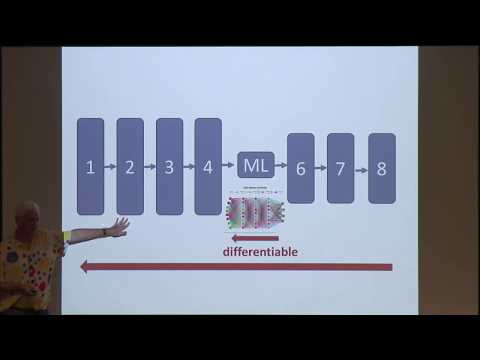
Peter Norvig (Google Director of Research) - As We May Program (May 2019)
Programming has undergone a remarkable transformation from early computers to modern devices, while challenges remain in making it universally accessible and incorporating natural language processing. Machine learning shifts the paradigm from traditional programming to an empirical model, extending beyond training to include data management and deployment.
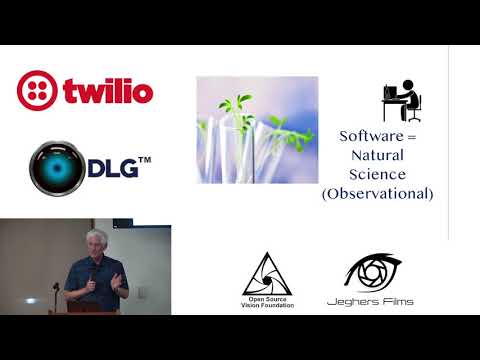
Peter Norvig (Google Director of Research) - As We May Program | Silicon Valley Deep Learning Group (Feb 2019)
Programming has dramatically evolved from strict logic instructions to assembling code from various sources, with ML revolutionizing the field and conversational AI promising a more natural user experience. The future of AI assistants relies on balancing innovation with ethical considerations, privacy, and security.
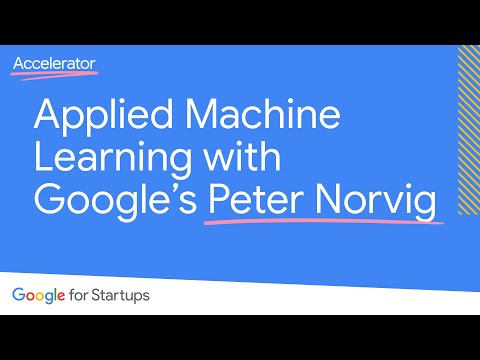
Peter Norvig (Google Director of Research) - Applied Machine Learning (Dec 2018)
Machine learning revolutionizes industries by automating tasks, improving decision-making, and uncovering patterns and correlations in data, but faces challenges in data acquisition, integration with existing systems, and balancing automation with human involvement.
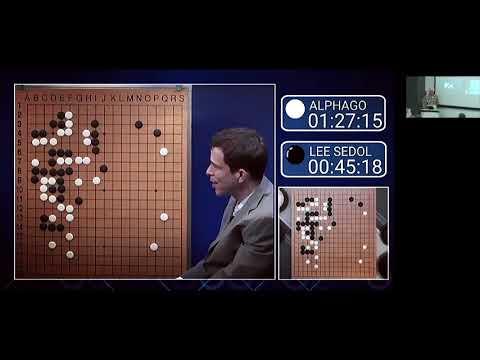
Peter Norvig (Google Director of Research) - Machine Learning and AI, Wonderfest Science Event (Jun 2018)
Machine learning has revolutionized software development, shifting from deterministic coding to a probabilistic approach with implications for creativity and data-driven development. Despite advancements, challenges in interpretability, data quality, privacy, security, and ethics must be addressed to harness machine learning's full potential.
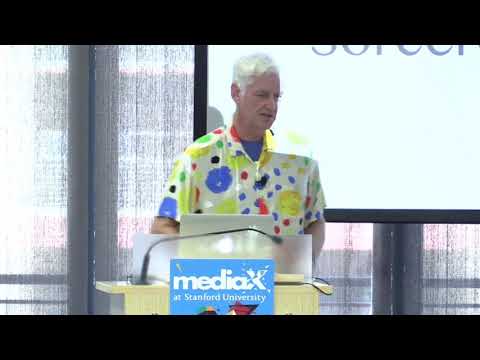
Peter Norvig (Google Director of Research) - Confidence, Trust and Certainty for Collaboration between Humans and Automated Systems (Feb 2018)
The emergence of machine learning in software development has shifted the focus from manual coding to machines learning from data, posing new challenges in debugging and communication between humans and AI systems. The integration of machine learning in software development raises ethical and practical issues, including concerns about privacy, security, fairness, and the optimization of AI systems.
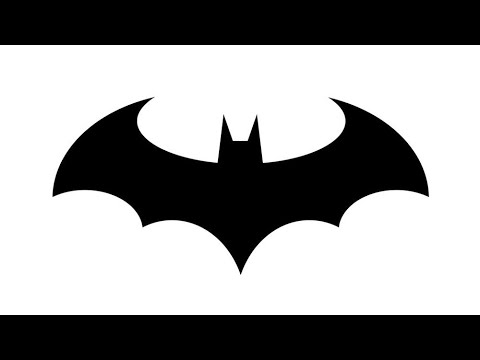
Peter Norvig (Google Director of Research) - Deploying Machine Learning Applications in the Enterprise (Sep 2017)
Machine learning, fueled by data abundance and computational power, is revolutionizing industries by enabling systems to learn from data and create solutions without explicit programming. Its potential to understand and interpret the world's essence promises breakthroughs in various fields, despite challenges like adversarial examples.
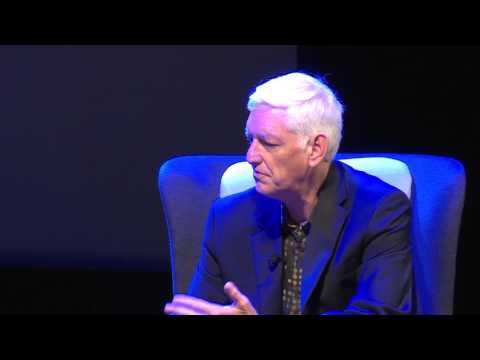
Peter Norvig (Google Director of Research) - Google's Approach to AI and Machine Learning (Jun 2017)
AI holds promise for revolutionizing sectors like transportation and enhancing daily life, but raises ethical concerns in warfare, job displacement, privacy, and autonomy. Rapid advancements in AI, especially deep learning, have led to breakthroughs in gaming, healthcare, and everyday applications, but limitations remain for tasks requiring long-term planning or reasoning.
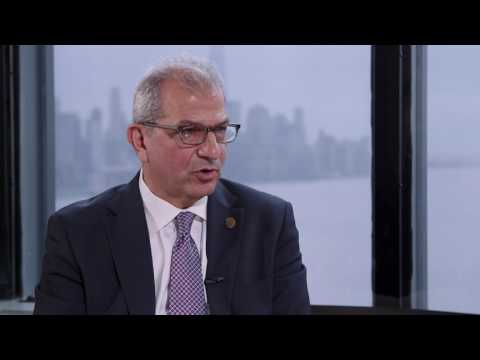
Peter Norvig (Google Director of Research) - Remarks at Stevens Institute of Technology (May 2017)
AI and ML have advanced due to increased data availability, faster computation, and algorithmic breakthroughs, transforming industries such as speech recognition, image recognition, and self-driving cars. Automation brought by AI and ML will likely augment jobs, increase societal productivity, but also lead to disruptions and job transformations.
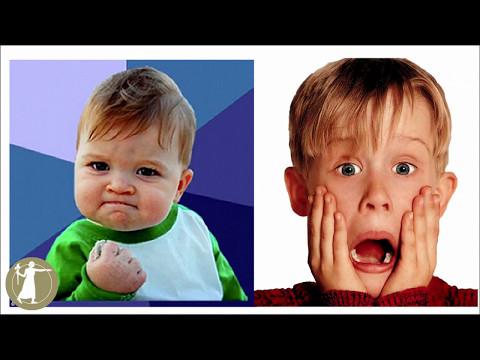
Peter Norvig (Google Director of Research) - Rethinking the Software Industry | National Academy of Sciences (May 2017)
Machine learning is revolutionizing programming, shifting from explicit instructions to machines learning from data, and raising ethical considerations due to black box models and data privacy concerns. Ethical AI development necessitates interdisciplinary collaboration, specialized teams, and new tools to analyze complex models and mitigate biases.
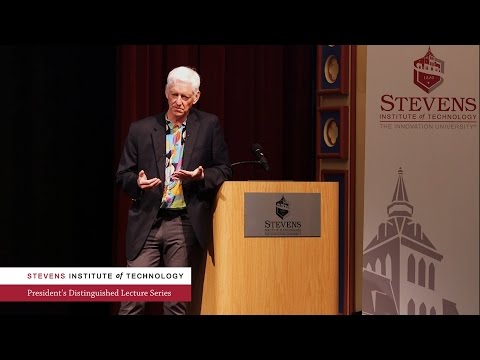
Peter Norvig (Google Director of Research) - Remarks at Stevens Institute of Technology (May 2017)
Machine learning's paradigm shift from traditional software development allows computers to learn from data and generate programs, offering unparalleled flexibility and speed in program development. Its applications range from natural language processing to computer vision, and it has the potential to revolutionize industries, but challenges like adversarial attacks and the unpredictable behavior of ML systems remain.
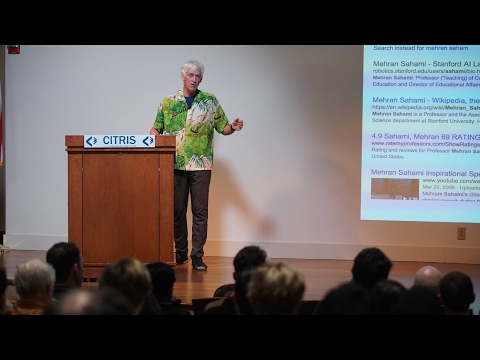
Peter Norvig (Google Director of Research) - Software Engineering with/and/for/by Machine Learning (Feb 2017)
Software engineering and machine learning convergence offers vast potential but presents technical and ethical challenges. Integrating ML into software engineering requires effective two-way communication, explainability, and careful consideration of risks and limitations.
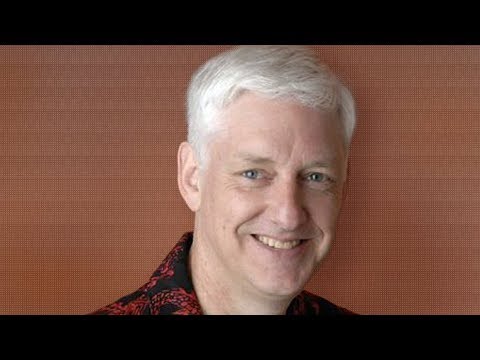
Peter Norvig (Google Director of Research) - Town Hall on A.I., Machine Learning, and More (Jan 2017)
AI is advancing rapidly due to increased computing power, more available data, and new techniques like deep learning. However, challenges remain in areas such as AI safety, ethical considerations, and the development of conversational AI systems that can understand context and reason effectively.
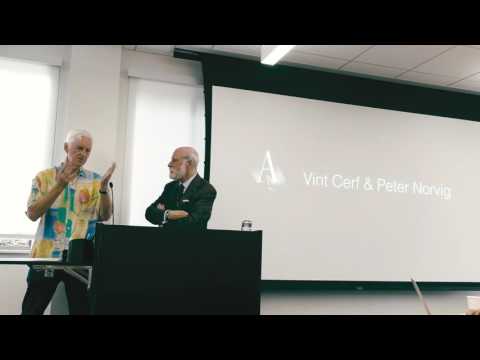
Peter Norvig (Google Director of Research) - The Future of Text Symposium (2016)
Advances in text analysis and research tools, such as Google Scholar, facilitate literature searches and hypothesis validation, while the evolution of writing software focuses on enhancing writability and fostering a flow state for writers. The symposium illuminated multifaceted challenges and opportunities in text preservation, interaction, and analysis, highlighting the dynamic nature of written communication and its role in shaping the future.
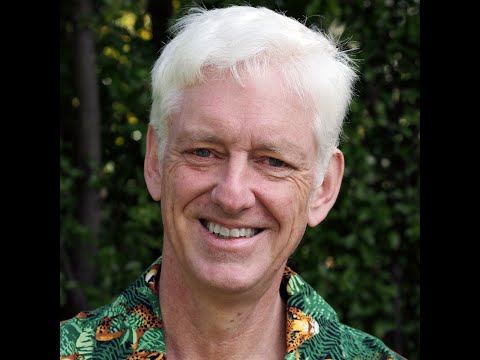
Peter Norvig (Google Director of Research) - Interfaces, Affordances, & Explanations for Machine Learning Systems (Oct 2016)
Machine learning and the evolution of human-computer interfaces bring transformative changes to our interactions with technology, posing challenges and ethical considerations. Embracing innovation, societal engagement, and responsible development is crucial for shaping the future of technology's integration into our lives.
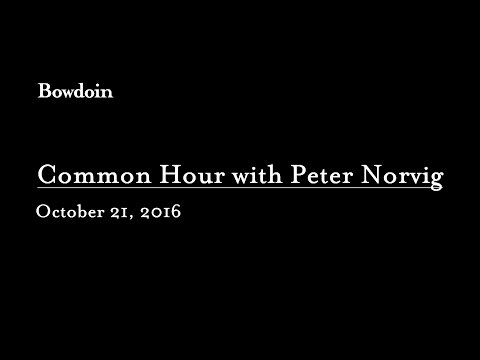
Peter Norvig (Google Director of Research) - The Challenges of Creating Software with Machine Learning (Oct 2016)
Machine learning revolutionizes software development by enabling data-driven decision-making, while natural language processing simplifies language processing and translation tasks.
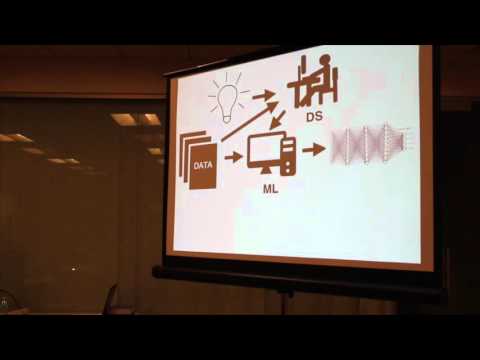
Peter Norvig (Google Director of Research) - Deep Learning and Understandability vs Software Engineering and Verification (Mar 2016)
The intersection of machine learning and software engineering is changing the landscape, offering new dimensions of efficiency and capability while also introducing challenges such as technical debt and data issues. Machine learning is revolutionizing software engineering, promising new efficiencies and capabilities, but also presenting challenges in tool development and data management.
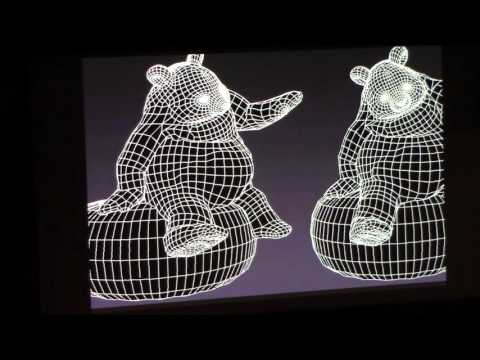
Peter Norvig (Google Director of Research) - 2015 LAST Festival (Oct 2015)
Machine learning has revolutionized software development by reducing explicit programming efforts and enabling new applications, but challenges such as adversarial examples and non-stationarity remain significant.
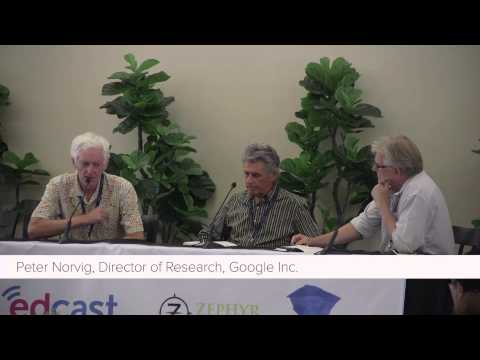
Peter Norvig (Google Director of Research) - The Future Learning Summit at Stanford (Jun 2015)
Technology is transforming education by fostering innovative learning experiences and enabling collaboration among educators, researchers, and students. The challenge lies in integrating technology effectively, addressing bottlenecks, and developing pedagogical approaches that cater to diverse learning styles.
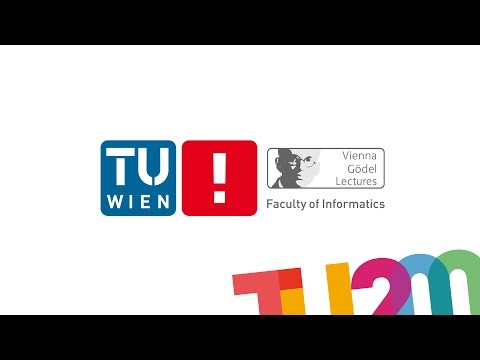
Peter Norvig (Google Director of Research) - How Computers Learn (Mar 2015)
Machine learning, a key pillar of AI, enables computers to learn from data and solve complex tasks without explicit programming, while AI faces challenges such as algorithmic inaccuracies, privacy issues, and the risk of false positives.
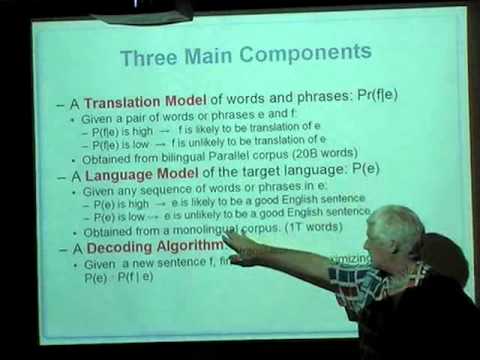
Peter Norvig (Google Director of Research) - Google Sets (Jun 2014)
Data-driven approaches have revolutionized computational linguistics, enabling statistical and probabilistic techniques to tackle language challenges and leading to advancements in statistical machine translation. Statistical machine translation utilizes statistical analysis to translate languages, delivering high-quality translations without the need for linguistic expertise.
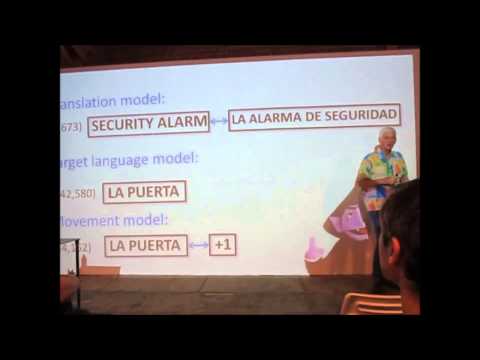
Peter Norvig (Google Director of Research) - AI talk at L.A.S.T. festival (Jun 2014)
The evolution of computing and AI has shifted from manual labor to knowledge-based tasks, and automation brings opportunities and challenges, such as streamlining processes and causing unemployment.
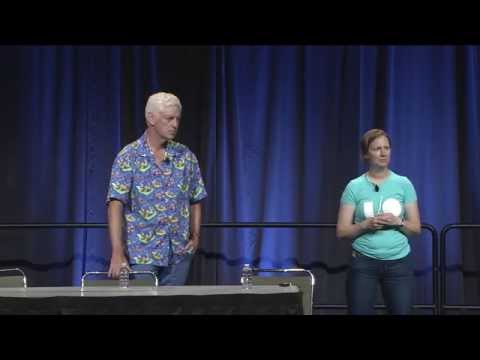
Peter Norvig (Google Director of Research) - Google I/O 2014 - Making sense of online course data (Jun 2014)
Data analysis of online education provides valuable insights for improving learning outcomes and identifying student difficulties. Self-evaluation methods can be effective in online courses with clear criteria and objectives, enhancing learners' metacognitive skills.
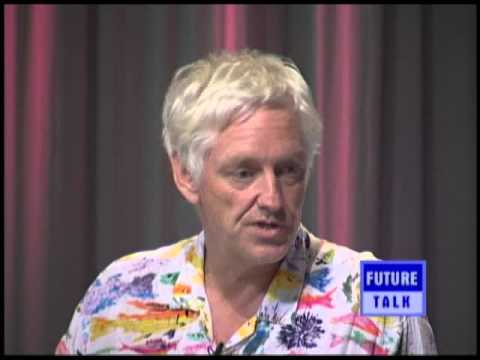
Peter Norvig (Google Director of Research) - Future Talk #32, Artificial Intelligence (Nov 2013)
Artificial intelligence (AI) has evolved from theoretical concepts to practical applications, significantly impacting various aspects of modern society, including language translation, image recognition, and autonomous vehicles. AI's ongoing challenges include enabling natural human interaction, replicating human capabilities, and addressing ethical and societal implications.
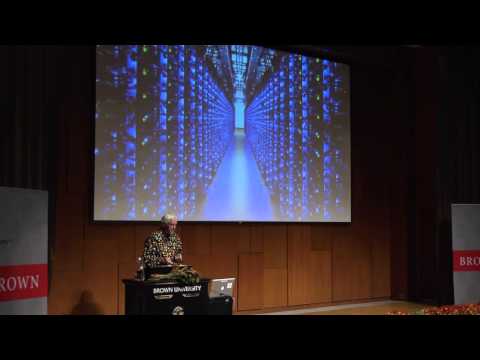
Peter Norvig (Google Director of Research) - Live and Learn (Oct 2013)
Technological advancements have led to a shift from manual labor to knowledge work and enabled computers to learn by imitating human actions. Data analysis has transformed historical research and machine translation, while ethical considerations are crucial in developing powerful technologies like AI.
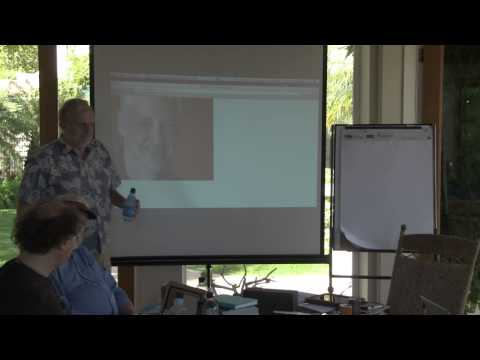
Peter Norvig (Google Director of Research) - Everything is Miscellaneous (Jul 2013)
Technological advancements in information retrieval and medical informatics balance innovation with privacy concerns, emphasizing the collaboration between systems and users for optimal interpretation and utilization. Machine learning in healthcare offers breakthroughs but faces challenges due to data biases and the need for theoretical grounding.
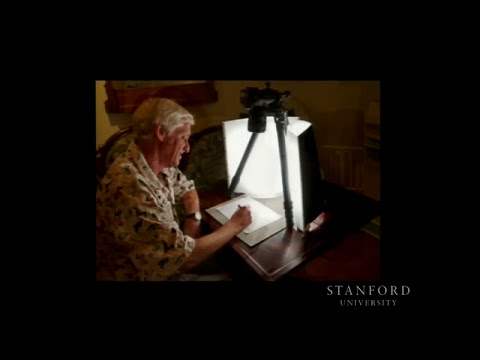
Peter Norvig (Google Director of Research) - "Online Education (Dec 2012)
Online learning has undergone a transformation, driven by shifts in methodologies and challenges, leading to innovative approaches like personalized learning and AI-powered tutoring. Online education offers flexibility, personalized learning, and problem-solving opportunities, but faces challenges in verification, cheating prevention, and credentialing.
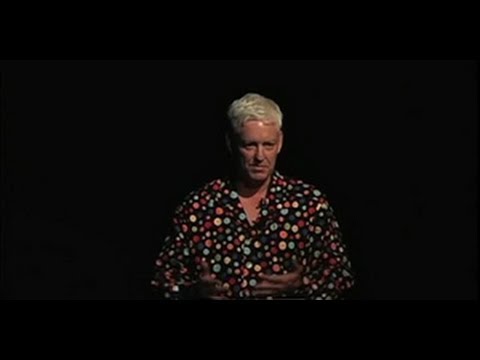
Peter Norvig (Google Director of Research) - Practice Makes Perfect | Santa Fe Institute (Apr 2012)
Data-driven approaches in AI, exemplified by Peter Norvig's work, have revolutionized image and text modeling, leading to qualitative improvements in AI tasks. Extensive datasets have transformed AI, enabling advanced image resizing, image search, and natural language processing.
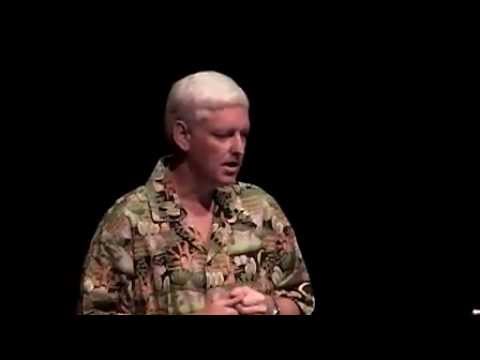
Peter Norvig (Google Director of Research) - The History and Future of Technological Change (Feb 2012)
Technological predictions are often inaccurate due to inherent uncertainty and political ideologies, while incremental progress is achieved through collective effort, culture, and data-driven AI advancements.
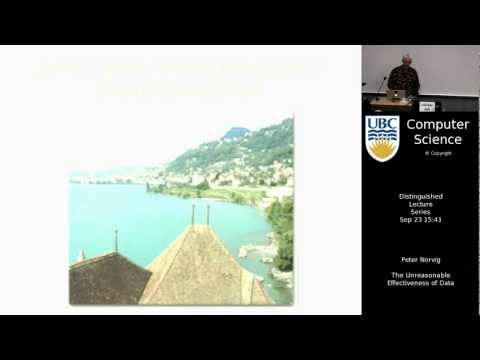
Peter Norvig (Google Director of Research) - The Unreasonable Effectiveness of Data (Oct 2011)
Mathematical principles, statistical models, and linguistic applications converge to enhance problem-solving, natural language processing, and machine translation. Data quantity and quality are vital for accurate language models, which benefit from the integration of supervised and unsupervised learning techniques.
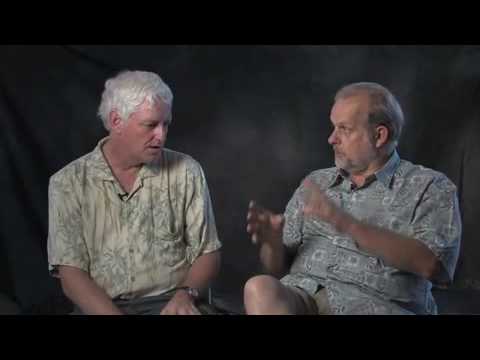
Peter Norvig (Google Director of Research) - Good Ancestors Principle workshop (May 2010)
Peter Norvig's contributions to AI, programming languages, and Google's search algorithms have left a lasting impact on the tech industry, while Google continues to innovate in search, AI, and renewable energy, facing challenges in data aggregation and trust.
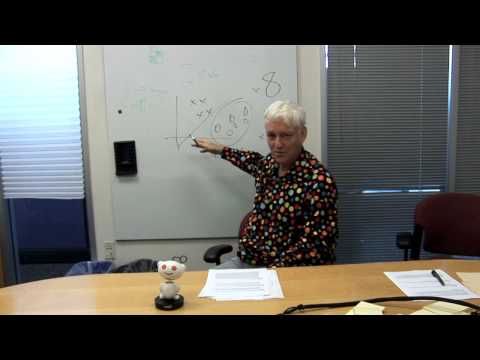
Peter Norvig (Google Director of Research) - reddit.com Interviews Peter Norvig (Feb 2010)
Google utilizes linear separators in complex machine learning tasks and emphasizes integrating research into practical applications, creating a forward-thinking and innovative environment in technology and organizational culture.
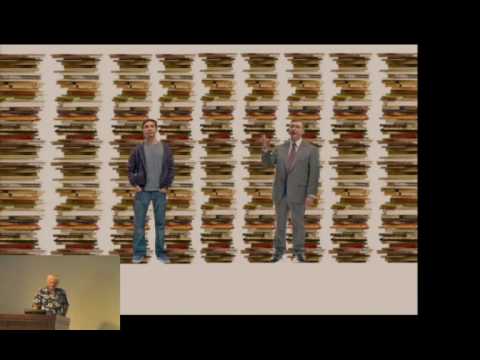
Peter Norvig (Google Director of Research) - Innovation in Search and Artificial Intelligence (Sep 2009)
Data-driven techniques in theory, image processing, and machine learning are outperforming complex algorithms, leading to advancements in AI and shaping the future of technology. Harnessing extensive datasets can enhance algorithmic capabilities and tackle real-world challenges, highlighting the potential and responsibilities of this rapidly evolving field.
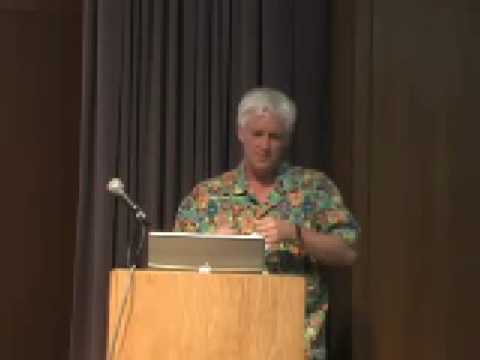
Peter Norvig (Google Director of Research) - Startup School 2008 (Apr 2008)
Startups can leverage data and machine learning to refine products and strategies in real-time, leading to improved accuracy and efficiency. Agile development and continuous evaluation ensure adaptability and growth in a rapidly changing market.
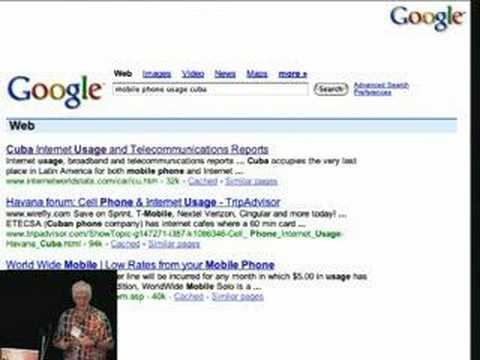
Peter Norvig (Google Director of Research) - The Future of Search (Nov 2007)
Google's Ice Cream Cone Theory categorizes search queries based on available information, ranging from abundant to scarce, requiring different retrieval strategies. Google employs various techniques, including query expansion and machine learning, to improve search accuracy and handle specialized queries.
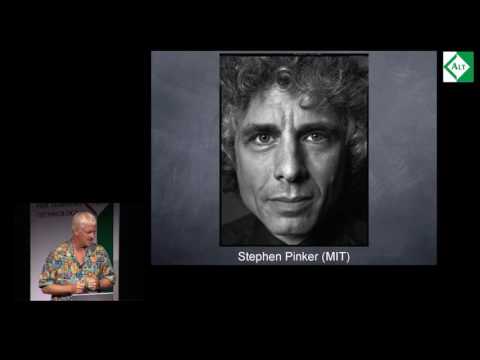
Peter Norvig (Google Director of Research) - Learning in an Open World (Sept 2007)
Technology offers opportunities to improve education by addressing challenges like Bloom's Two-Sigma Problem and revolutionizing conventional teaching methods, but it also brings challenges like information overload and the need for scalable alternatives to one-on-one tutoring.
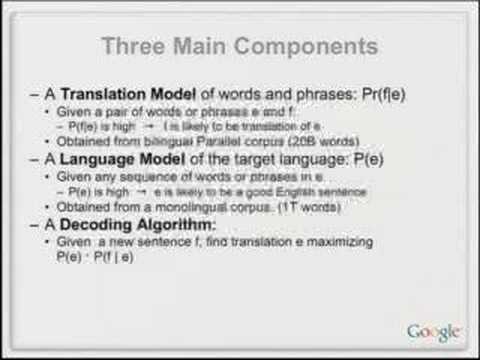
Peter Norvig (Google Director of Research) - Theorizing from Data (Jun 2007)
Data's evolution from scarce to abundant has transformed AI, NLP, and search technologies, with Google's trillion-word corpus and innovative tools showcasing its transformative power. Statistical methods and data-driven approaches have revolutionized language processing and translation, while data's abundance enables ongoing advancements in AI and NLP.