Lukasz Kaisar
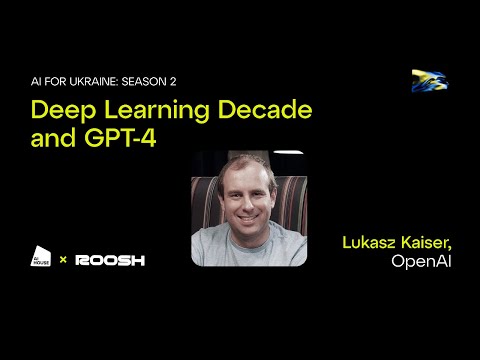
Lukasz Kaisar (OpenAI Technical Staff) - Deep Learning Decade and GPT-4 (Nov 2023)
Deep learning pioneer Lukasz Kaiser's journey highlights advancements in AI capabilities, challenges in ensuring truthfulness and ethical alignment, and the potential for AI to enhance human capacities. The AI for Ukraine Initiative showcases the power of AI to address global issues.
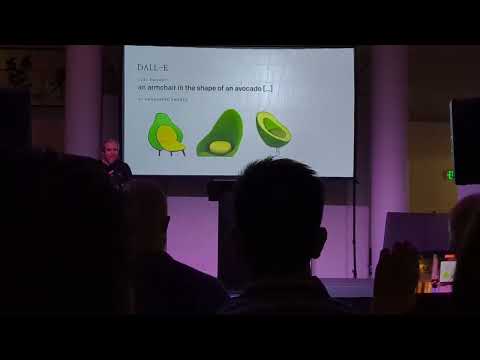
Lukasz Kaisar (OpenAI Technical Staff) - GPT-4 and Beyond (Sep 2023)
Neural networks have undergone a transformative journey, with the Transformer model revolutionizing language processing and AI systems shifting from memorization to genuine understanding. Advancements like chain-of-thought prompting and the move beyond gradient descent suggest a future of AI with autonomous learning and reasoning capabilities.
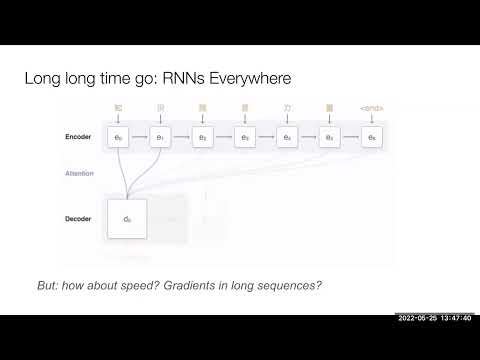
Lukasz Kaisar (OpenAI Technical Staff) - Basser Seminar at University of Sydney (Aug 2022)
Transformers have revolutionized AI, enabling advancements in NLP, image generation, and code generation, but challenges remain in scaling and improving data efficiency. Transformers have shown promise in various tasks beyond NLP, including image generation, code generation, and robotics, but data scarcity and computational complexity pose challenges.
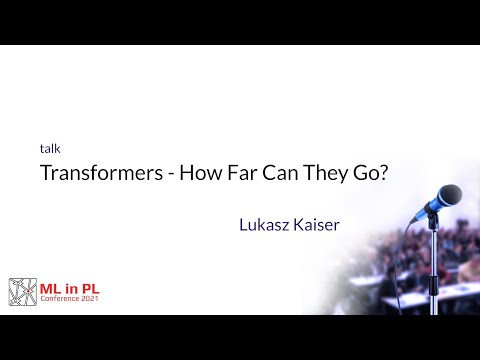
Lukasz Kaisar (OpenAI Technical Staff) - Transformers - How Far Can They Go? (Mar 2022)
Transformers, a novel neural network architecture, have revolutionized NLP tasks like translation and text generation, outperforming RNNs in speed, accuracy, and parallelization. Despite computational demands and attention complexity, ongoing research aims to improve efficiency and expand transformer applications.
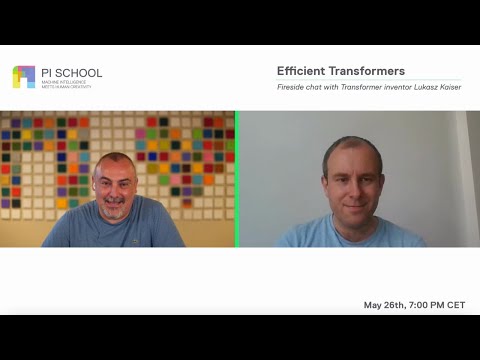
Lukasz Kaisar (Google Brain Research Scientist) - A new efficient Transformer variant (May 2021)
Transformers have revolutionized NLP and AI with their speed, efficiency, and performance advantages, but face challenges in handling extremely long sequences and computational cost. Ongoing research and innovations are expanding their applicability and paving the way for even more advanced and diverse applications.
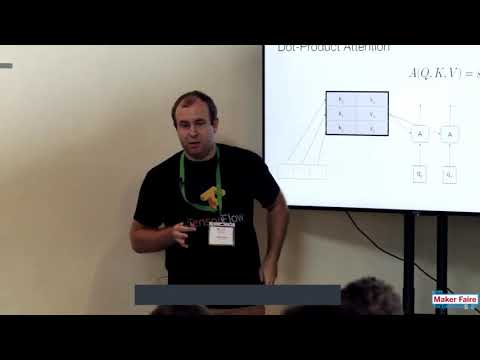
Lukasz Kaisar (Google Brain Research Scientist) - Attention is all you need. Attentional neural network models - Maker Faire Rome (Dec 2020)
Neural networks and attention mechanisms have revolutionized natural language processing, particularly machine translation, with the Transformer model showing exceptional results in capturing relationships between words and improving translation accuracy. The Transformer model's multitasking capabilities and potential for use in image processing and OCR indicate a promising future for AI applications.
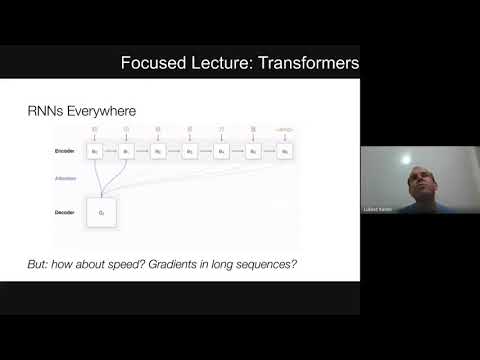
Lukasz Kaisar (Google Brain Research Scientist) - Day 4 (Jul 2020)
Transformer models revolutionized NLP by parallelizing processing and employing the self-attention mechanism, leading to faster training and more efficient long-sequence modeling. Transformers' applications have expanded beyond NLP, showing promise in fields like time series analysis, robotics, and reinforcement learning.
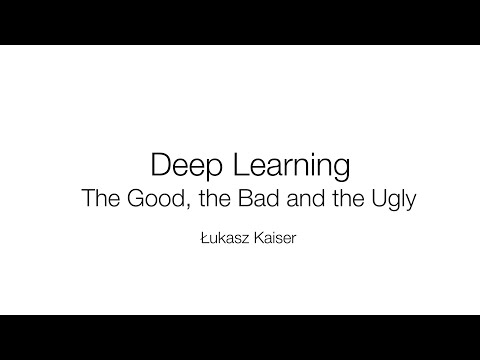
Lukasz Kaisar (Google Brain Research Scientist) - "Deep Learning (Aug 2018)
Deep learning revolutionizes NLP by unifying tasks under a single framework, enabling neural networks to learn end-to-end without explicit linguistic programming. Deep learning models excel in text generation, capturing long-range dependencies and producing fluent, coherent sentences, outshining traditional methods in machine translation and parsing.
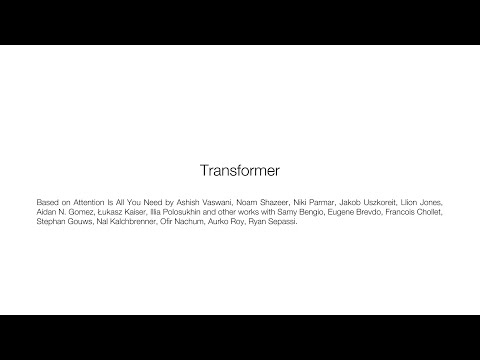
Lukasz Kaisar (Google Brain Research Scientist) - "Deep Learning (Aug 2018)
Transformers revolutionized language processing by utilizing attention mechanisms and enhanced sequence generation capabilities, leading to breakthroughs in translation and various other tasks. Attention mechanisms allow models to focus on important aspects of input sequences, improving performance in tasks like translation and image generation.
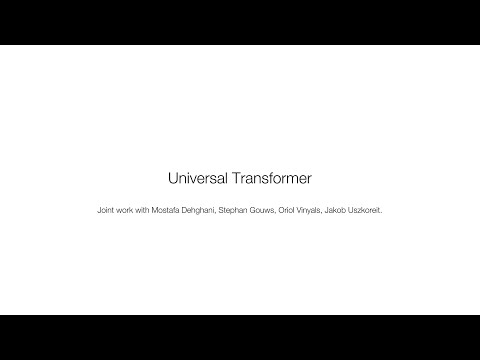
Lukasz Kaisar (Google Brain Research Scientist) - "Deep Learning (Aug 2018)
The introduction of Transformers and Universal Transformers has revolutionized AI, particularly in complex sequence tasks, enabling efficient handling of non-deterministic functions and improving the performance of language models. Multitasking and unsupervised learning approaches have further enhanced the versatility and efficiency of AI models in various domains.
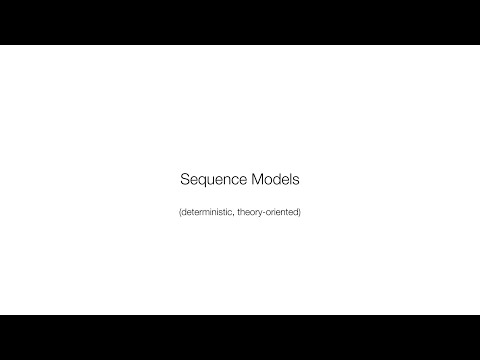
Lukasz Kaisar (Google Brain Research Scientist) - "Deep Learning (Aug 2018)
Neural networks have evolved from simple models to complex architectures like RNNs and NTMs, addressing challenges like training difficulties and limited computational capabilities. Advanced RNN architectures like NTMs and ConvGRUs exhibit computational universality and perform complex tasks like long multiplication.
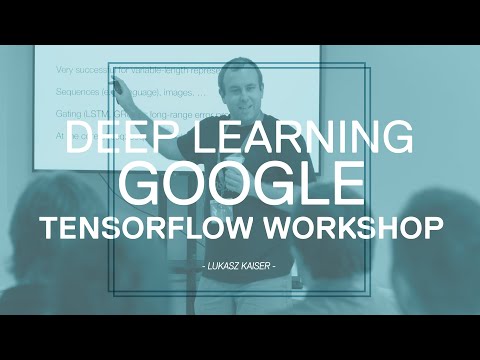
Lukasz Kaisar (Google Brain Research Scientist) - Deep Learning TensorFlow Workshop (Oct 2017)
TensorFlow, a versatile machine learning framework, evolved from Google's DistBelief to address computational demands and enable efficient deep learning model development. TensorFlow's graph-based architecture and mixed execution model optimize computation and distribution across various hardware and distributed environments.
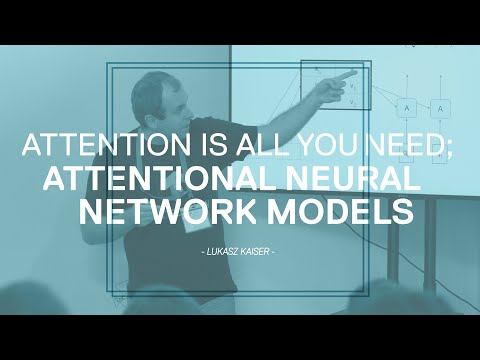
Lukasz Kaisar (Google Brain Research Scientist) - Attention is all you need; Attentional Neural Network Models (Oct 2017)
Transformer models, with their attention mechanisms, have revolutionized natural language processing, enabling machines to understand context and generate coherent text, while multitasking capabilities expand their applications in data-scarce scenarios.